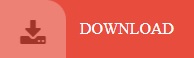

When imaging data are the primary input for a machine learning model, one of the most powerful and commonly used subtypes of machine learning is a convolutional neural network (CNN), which employs hierarchical learning to assimilate the complexities of images and spatially inconsistent features.ĬNNs decompose image inputs into more basic visual features such as lines and edges, which can themselves combine to form shapes that in turn can combine to form groups of shapes. Poor-quality images were excluded and the UWF images were cropped to a standardised region of interest to avoid or remove artifacts, yielding high-fidelity data. The images were manually assessed for quality prior to adding to the input dataset. The ground truth (AD or cognitively normal) was determined by a team of expert neurologists participants with underlying conditions, which could bias the detection accuracy, were excluded from the study. In addition to the raw images, subjects’ age, sex and visual acuity as well as quantitative metrics of retinal microvasculature and structure from the OCT and OCTA scans were included. 2 The model was able to distinguish those with symptomatic AD from cognitively normal controls, serving as a proof of concept for using the retina as a “window to the brain” and exhibiting promise for artificial intelligence (AI) as a powerful tool in the diagnosis of AD.įor this study, the iMIND team collected and used the following types of retinal images: ganglion cell-inner plexiform layer (GC-IPL) thickness colour maps from optical coherence tomography (OCT), superficial capillary plexus en face 3 × 3 mm and 6 × 6 mm OCT angiography (OCTA) images centred on the fovea, ultra-widefield (UWF) scanning laser ophthalmoscopy (SLO) colour fundus photos, and UWF SLO fundus autofluorescence images. To test this possibility, the Eye Multimodal Imaging in Neurodegenerative Disease (iMIND) lab at Duke University applied modern machine learning techniques to retinal images and clinical patient data, as shared in their recent publication in the British Journal of Ophthalmology. With the identification of microvascular and neurosensory structural alterations in the retinas of individuals with AD, non-invasive retinal imaging may be an adjunctive or even an alternative means of detecting AD early.
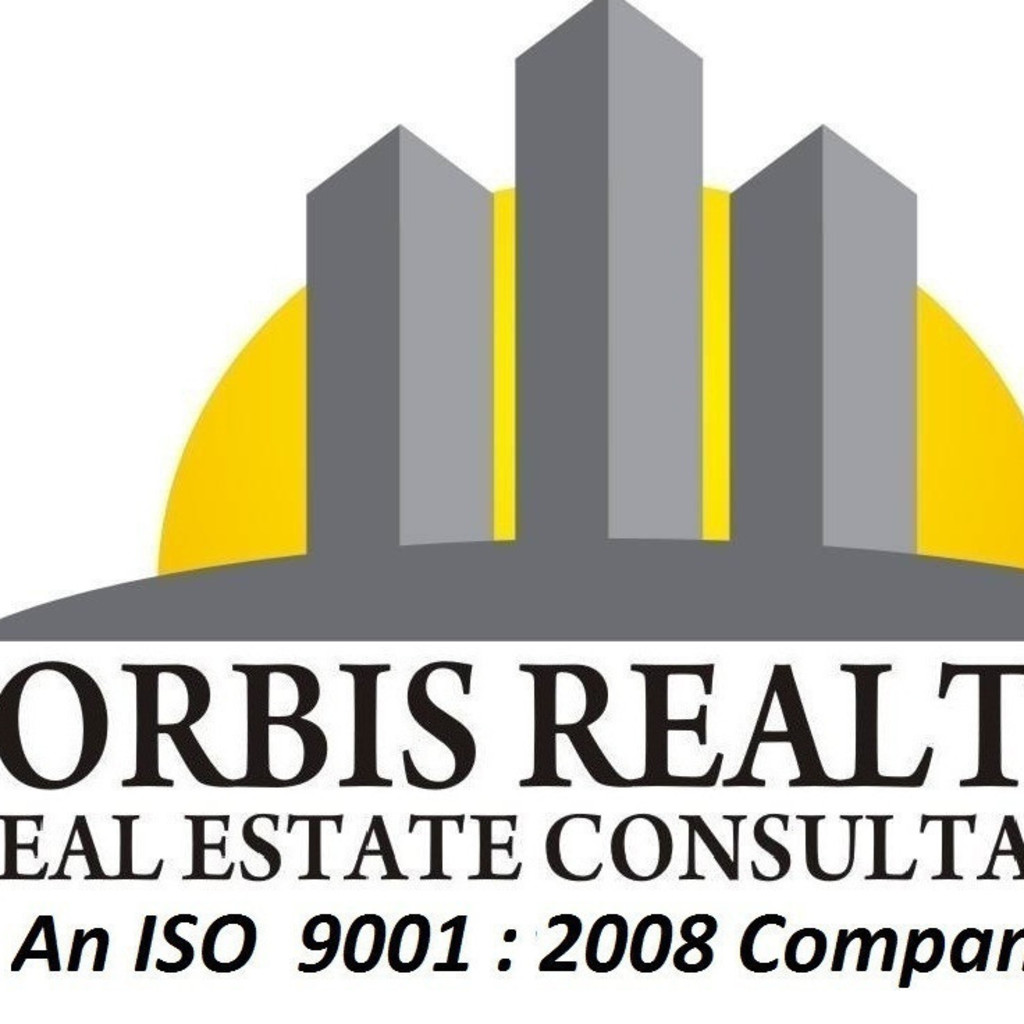
A common neurodegenerative disorder, Alzheimer disease (AD) is typically diagnosed symptomatically because of the high cost and invasiveness of alternative methods like positron emission tomography scans and lumbar punctures.
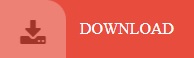